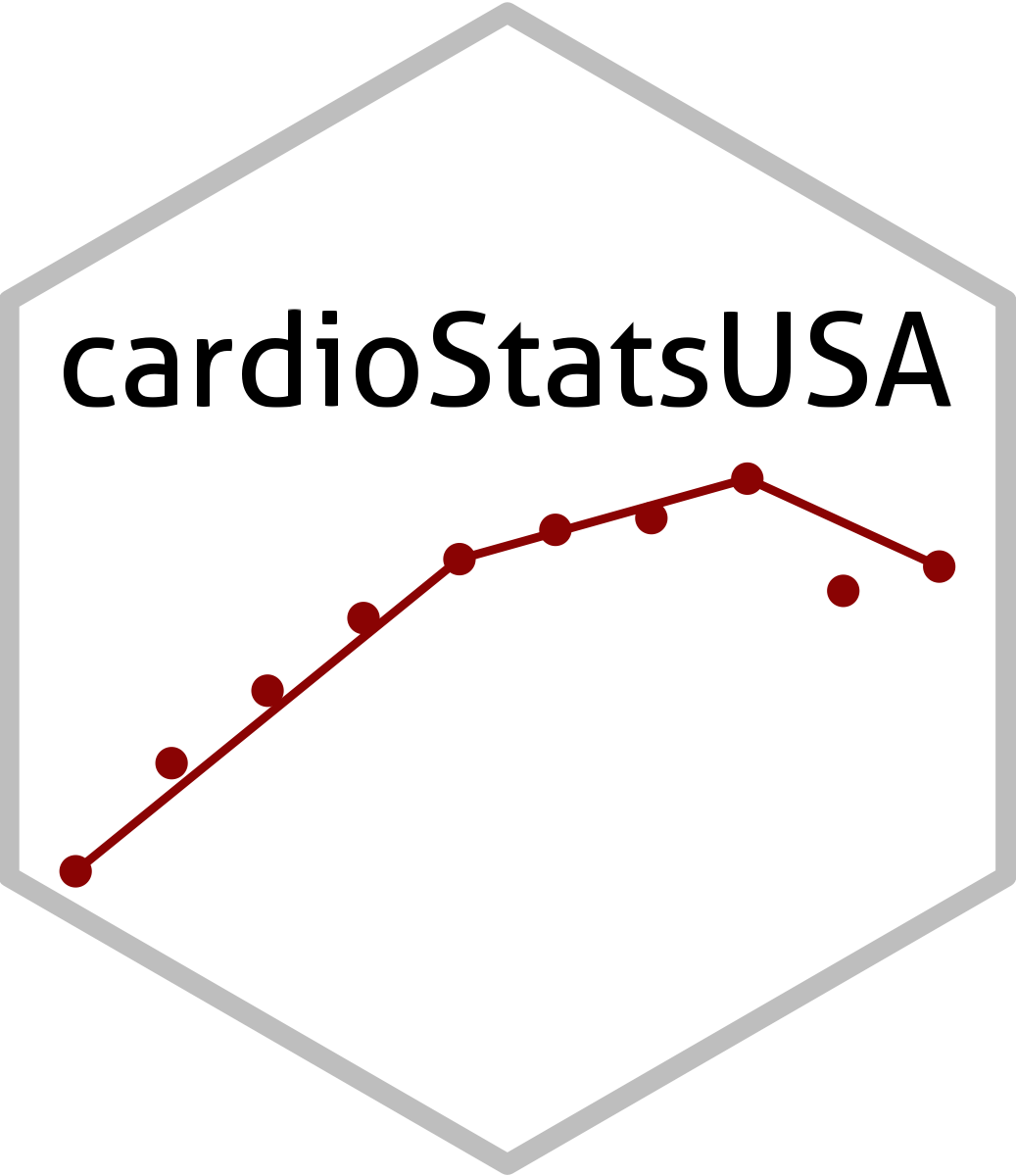
Generate summaries of NHANES
nhanes_summarize.Rd
The description should include
what is NHANES
Who is included in the data to be summarized
How is the summary computed
Usage
nhanes_summarize(
data,
key,
outcome_variable,
outcome_quantiles = NULL,
outcome_stats = NULL,
group_variable = NULL,
group_cut_n = NULL,
group_cut_type = NULL,
stratify_variable = NULL,
time_variable = "svy_year",
time_values = NULL,
pool = FALSE,
subset_calls = list(),
standard_variable = "demo_age_cat",
standard_weights = NULL,
simplify_output = TRUE
)
Arguments
- data
[data.frame] A set of NHANES data with one row per survey participant and one column per variable. See nhanes_data for more details See Details for specific requirements. See nhanes_data for an example.
- key
[data.frame] A data set with one row per variable and with columns that describe the variable. See nhanes_key for more details See Details for specific requirements. See nhanes_key for an example.
- outcome_variable
[character(1)] The name of the outcome variable to be summarized.
- outcome_quantiles
[numeric(1+)] The quantiles to be summarized for a continuous outcome. The default is
c(0.25, 0.50, 0.75)
. For example,outcome_quantiles = c(.5)
will compute the 50th percentile (i.e., the median)outcome_quantiles = c(.25, .5, .75)
will compute the 25th, 50th, and 75th percentile.outcome_quantiles = seq(.1, .9, by = .1)
will compute every 10th percentile, except for the 0th and 100th
- outcome_stats
[character(1+)]
The statistics that should be computed. Multiple statistics may be requested. Valid options depend on the type of outcome to be summarized. For continuous outcomes, valid options include
'mean': estimates the mean value of the outcome
'quantile': estimates 25th, 50th, and 75th percentile of the outcome.
For categorical outcomes, valid options include
'percentage': estimates the prevalence of the outcome
'percentage_kg': estimates the prevalence and uses Korn and Graubard's method to estimate a 95% confidence interval
'count': estimates the number of US adults with the outcome.
- group_variable
[character(1)] The name of the group variable. See Details for a description of the group variable and the stratify variable.
- group_cut_n
[integer(1)] The number of groups to form using the group variable. This is only relevant if the group variable is continuous, and can be omitted. Default is 3
- group_cut_type
[character(1)] The method used to create groups with the grouping variable. This is only relevant i fthe group variable is continuous, and can be omitted. Valid options are:
"interval": equal interval width, e.g., three groups with ages of 0 to <10, 10 to <20, and 20 to < 30 years.
"frequency": equal frequency, e.g., three groups with ages of 0 to <q, q to <p, and p to <r, where q, p, and r are selected so that roughly the same number of people are in each group.
- stratify_variable
[character(1)] the name of the stratify variable. See Details for a description of the group variable and the stratify variable.
- time_variable
[character(1)] The name of the time variable. The default,
svy_year
, corresponds to the variable innhanes_data
that indicates which 2 year NHANES cycle an observation was collected in.- time_values
[character(1+)] The time values that will be included in this design object. The default is to include all time values present in
data
. Valid options are:'most_recent'
: includes the most recent time value.'last_5'
: includes the 5 most recent time values.'all'
: includes all time values present indata
.You can also give a vector of specific time values, e.g.,
c("2009-2010", "2011-2012", "2013-2014")
, if these values are present in the time_variable column (they are fornhanes_data
).
- pool
[logical(1)] If
FALSE
(the default), results are presented for individual times, separately. IfTRUE
, data from each time value are pooled together. Note that only contiguous cycles should be pooled together, e.g., usingpool = TRUE
withtime_values = 'last_5'
is okay, but usingpool = TRUE
withtime_values = c("2009-2010", "2013-2014")
is not recommended (that would be a strange result to interpret).- subset_calls
[named list(n)]
the names of
subset_calls
are variable names, and the values are values of the variable to include in the subsetted data. For example,subset_calls = list("demo_gender" = "Women")
will subset the data to include rows wheredemo_gender
is equal to"Women"
. Multiple entries are allowed and collapsed with the logical&
operator. For example,subset_calls = list(demo_gender = "Women", bp_med_use = "Yes")
will subset the data to include rows wheredemo_gender
is equal to'Women'
ANDbp_med_use
is equal to"Yes"
- standard_variable
[character(1)]
The name of the variable used to create standardization groups. The default is to use
demo_age_cat
, which leads to age standardization.- standard_weights
[numeric(n)]
The proportionate weights for each group defined by the standard variable. The number of weights should equal the number of groups defined by
standard_variable
and all weights must be >0.- simplify_output
[logical(1)]
The type of output returned will be nhanes_design if
simplify_output
isFALSE
and adata.table
otherwise.
Value
if simplify_output
is TRUE
, a data.table
.
Otherwise, an nhanes_design object is returned.
Examples
nhanes_summarize(data = nhanes_data,
key = nhanes_key,
outcome_variable = "bp_sys_mean")
#> svy_year statistic estimate std_error ci_lower ci_upper n_obs
#> <fctr> <char> <num> <num> <num> <num> <int>
#> 1: 1999-2000 mean 122.7799 0.7232809 121.3623 124.1975 4694
#> 2: 2001-2002 mean 122.4941 0.4625177 121.5876 123.4006 5181
#> 3: 2003-2004 mean 122.6814 0.4827482 121.7352 123.6275 4836
#> 4: 2005-2006 mean 122.2579 0.4650055 121.3465 123.1692 5012
#> 5: 2007-2008 mean 121.5421 0.3779936 120.8013 122.2830 5664
#> 6: 2009-2010 mean 120.4879 0.4809511 119.5452 121.4305 6043
#> 7: 2011-2012 mean 121.6124 0.6464260 120.3454 122.8794 5334
#> 8: 2013-2014 mean 121.4265 0.3198856 120.7996 122.0535 5692
#> 9: 2015-2016 mean 123.3559 0.4613174 122.4517 124.2600 5551
#> 10: 2017-2020 mean 123.1297 0.3709403 122.4027 123.8567 8010
#> unreliable_status unreliable_reason review_needed review_reason
#> <lgcl> <char> <lgcl> <char>
#> 1: FALSE <NA> FALSE <NA>
#> 2: FALSE <NA> FALSE <NA>
#> 3: FALSE <NA> FALSE <NA>
#> 4: FALSE <NA> FALSE <NA>
#> 5: FALSE <NA> FALSE <NA>
#> 6: FALSE <NA> FALSE <NA>
#> 7: FALSE <NA> FALSE <NA>
#> 8: FALSE <NA> FALSE <NA>
#> 9: FALSE <NA> FALSE <NA>
#> 10: FALSE <NA> FALSE <NA>